2021-2023
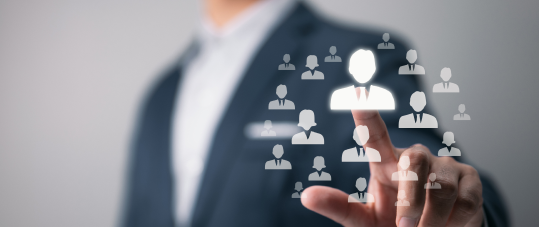
Unsupervised crowdsourcing with cost and accuracy guarantees
The crowdsourcing platform is assumed to be divided into multiple classes, based on workers skill, experience, etc. We consider the problem of cost optimal utilization of a crowdsourcing platform for binary, unsupervised classification of a collection of items, given a prescribed error threshold.
Faculty associated
Prof. N. Hemachandra (IEOR) and
Prof. Jayakrishnan Nair (EE)
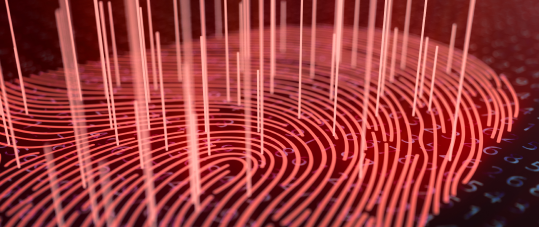
Automating Threat Detection and Response in Linux Endpoints
Endpoint Detection and Response EDR is an advanced technology for the detection and prevention of attacks on cyberinfrastructure. It is an integrated security solution that combines realtime continuous monitoring and collection of endpoint data with rules-based automated response and analysis capabilities.
Faculty associated
Prof. Manjesh K. Hanawal (IEOR)
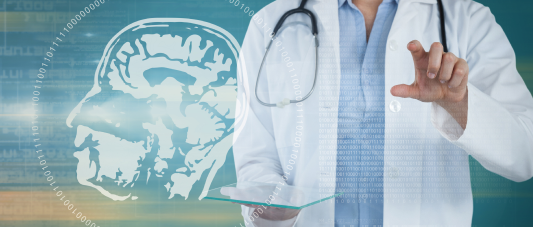
3D Medical Image data synthesis for classification and segmentation using deep generative techniques abeling 3D medical images
Typical prediction tasks using 3D medical images like classification and segmentation using deep neural networks require large amounts of labeled training data. In this project, we will develop novel variants of deep networks e.g. generative adversarial networks GANs, variational encoders VAEs to synthesize 3D medical image data for classification and segmentation tasks.
Faculty associated
Prof. P. Balamurugan (IEOR)